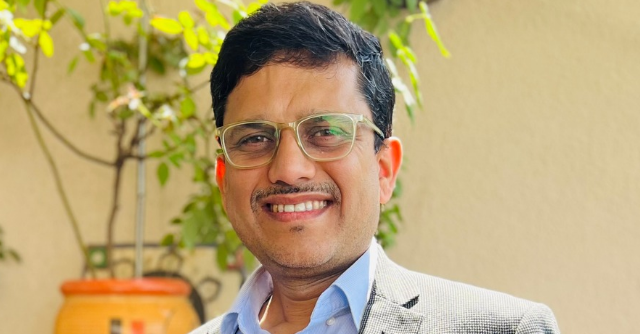
New Delhi, April 22 -- California-headquartered R Systems is a global technology and analytics services company with over 30 years of experience helping enterprises accelerate digital transformation. It has a strong focus on data, Artificial Intelligence (AI), and next-gen technologies, and partners with clients across industries, including financial services, healthcare, telecom, and technology.
TechCircle interviewed Neeraj Nayan Abhyankar, the vice president of data and AI at the company. Edited excerpts:
What are the major projects R Systems working on this year?
When we look at the evolution of the data and analytics space over the past few years, it's fascinating to see how it has matured. Up until around 2020-2022, the focus was primarily on descriptive analytics. Then came the rise of data science and predictive analytics, which drove much of the conversation during that period. In 2023, we witnessed a significant shift with the emergence of generative AI, and now, the spotlight has moved to agent-based AI.
Our team has kept pace with these evolving trends-and, in many cases, is leading from the front. For example, we're developing agent-based automated appointment scheduling solutions for our healthcare customers. For HR tech clients, we've built HR buddy systems that support intent-based delegation and trigger specialised agents to handle queries and tasks.
Of course, all of this is on the consumption side, how insights are applied in real-time. My own focus is on the foundation: data and analytics. Data remains at the heart of everything we do. Our aim is to ensure that it delivers measurable value for our customers, whether that's enhancing end-user experience, driving cost savings, or improving overall efficiency.
While data is central to evolving AI systems and solutions, many industry observers and experts have flagged the lack of quality data. How do you deal with this challenge?
Traditionally, we've always ensured that any data being consumed is backed by a data owner, someone who certifies and validates its accuracy and reliability. Such a governance model has worked well in the past. However, with the advent of generative AI and large language models (LLMs), the scale of data and its usage have expanded significantly, making traditional validation models insufficient.
To address this, we've built a robust process for checks and balances across the lifecycle of these solutions.
We follow a two-pronged approach. First, when we work with publicly available LLMs in partnership with vendors, we adopt a retrieval-augmented generation (RAG) approach. This is done in the context of the specific customer for whom we're building the solution. The process involves multiple layers of internal validation to ensure output quality.
Second, we make sure that the contextual data, which is sourced from the customer's environment, must be certified by someone with authority within that domain. This ensures that the foundational layer of knowledge feeding the AI is both trusted and accurate.
For most implementations, we recommend having an "expert in the loop" or a human-in-the-loop setup. This ensures oversight and enhances confidence in the outcomes of these AI systems.
Can synthetic data be an effective way to deal with the shortage of quality real data?
Synthetic data definitely has its place, and it ties back to the earlier point we discussed. It's a great starting point for testing, especially in the initial phases of sensitive projects where accessing real data on day one isn't feasible. Synthetic data helps us get the machinery running. It allows us to begin initial tests, validate technical flows, and prepare the foundation.
However, synthetic data alone doesn't complete the journey. For a project to be truly production-ready, it must be tested, touched, and approved by the actual end users using real data, especially in the final rounds of testing. That's when we know we've done the complete job. This approach becomes critical in regulated industries like financial services or telecom, where we have to be extremely conservative and precise.
What are some of the Agentic AI projects that R Systems is working on?
We began our experimentation with agent-based AI around the second half of 2024. Initially, it was all about trying out ideas, running pilots. But toward the end of 2024, we started pushing these into production. Today, around 10% (about 100) of our case studies involve agentic AI in some form.
There is an emerging trend that we have observed among our customers that they want to move beyond just rule-based or traditional AI; they are seeking more autonomy with systems that can stitch steps together, adapt dynamically, and operate independently. This is driving the next wave of agentic AI use cases.
We're also seeing traction in document-heavy domains like healthcare, insurance, and HR, where we've automated tasks like classification and next-step execution. Now, we're expanding these systems with richer data and broader task coverage to reduce manual effort and let teams focus on high-value work.
You have been quick to spot emerging AI trends. How do you balance the urgency to deploy with ensuring long-term relevance without getting carried away by the hype?
Early in my career, I worked extensively with financial services clients, where we followed a cautious and methodical approach-waiting until everything was fully settled before moving forward. But as my career evolved, I was exposed to other domains where there's more agility and openness to experimentation.
At R Systems, our clientele is a healthy mix across sectors, which allows us to balance both worlds.
We work with a significant number of independent software vendors (ISVs) and tech clients, we're often invited to participate early in experimental initiatives. That's a real advantage.
One such client was facing technical debt with legacy systems and was evaluating whether to adopt LLMs, rule-based approaches, or off-the-shelf product solutions. We worked with them to co-analyse the landscape. Eventually, we embedded agentic AI capabilities into their system as they transitioned to newer technologies.
We also conduct internal experimentation. We have ongoing academic collaborations and a team that actively tracks developments in both classical and modern AI. While we remain excited about emerging technologies, we don't chase trends blindly. Our approach is nimble and grounded in practical value rather than hype.
Tell us about R Systems' AI and data team.
Our team spans the entire data and AI spectrum, covering data engineering, BI, classical AI, generative AI, and agentic AI. Today, we have over 200 data and AI professionals, all cross-trained across these domains.
Currently, around 50% of this team is fully dedicated to AI in some form, and that number is rapidly growing. We're seeing strong demand across geographies, particularly in the AI space. AI often becomes the entry point for many engagements. Customers come to us with a specific AI use case, only for us to discover underlying data challenges or legacy data models that also need attention. Our presence is global. While India remains our innovation and delivery hub, we have teams operating across the US, Europe, and APAC.
Published by HT Digital Content Services with permission from TechCircle.